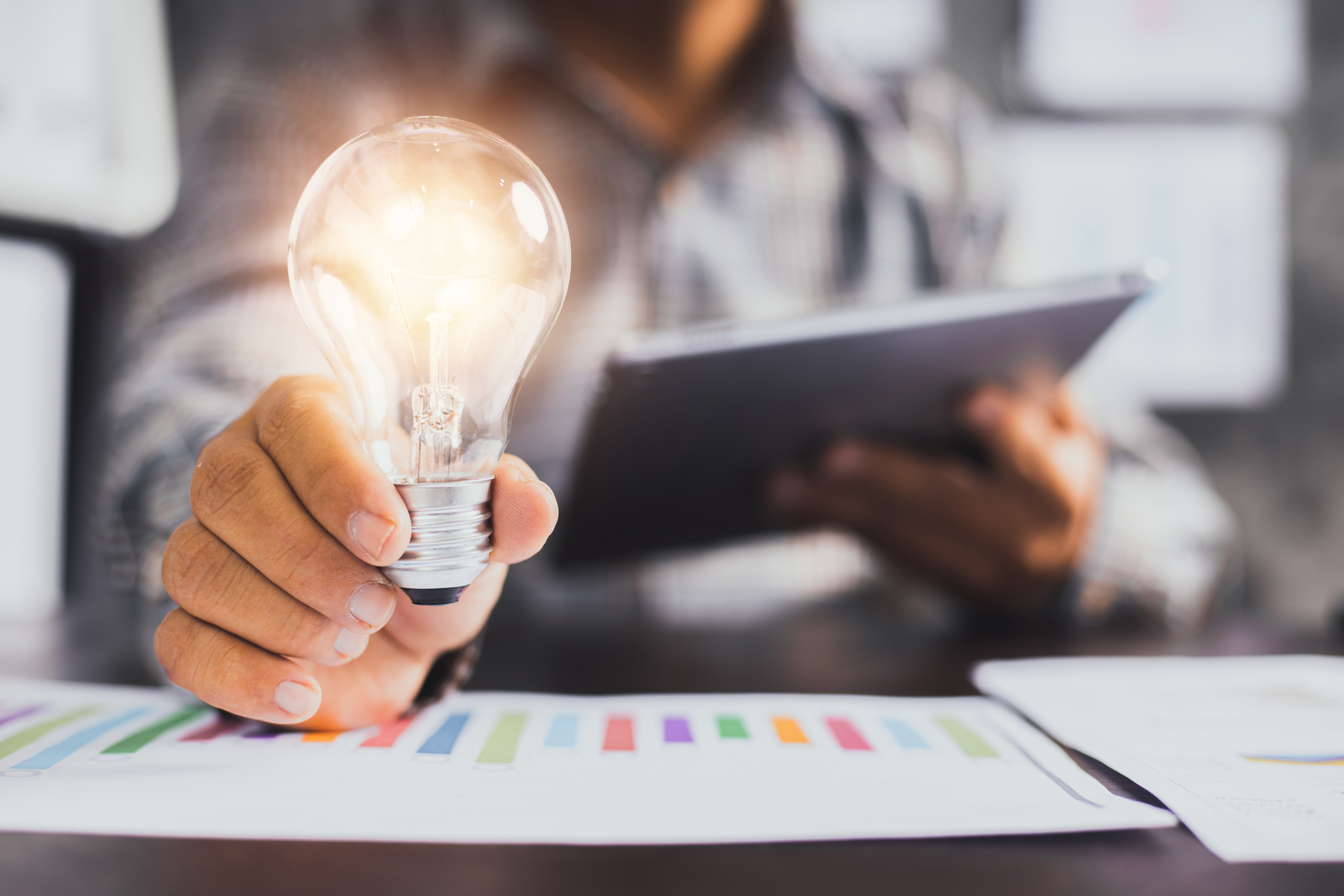
Is It Time to Dive Into a Data Lake?
by Neil Leyshock
Every organization has data, and the volume and variety of data is continuously increasing. But where do you store all this data? If your organization is like many others, you may be trying to decide between the more organized structure of a data warehouse or the raw form of a data lake.
The good news is you do not have to choose one over the other.
Many sources of information are used to help drive different aspects of a business. At times, separate streams of data are brought together to provide greater insight. However, data often resides in silos, where it is analyzed by the business unit responsible for the information, summarized, and shared as a set of results to the rest of the organization. A great example of this is marketing research data. Surveys are carefully crafted to provide insight around key business questions, but require upfront knowledge of the right questions to ask and the right attributes to rate. More and more, organizations seek to link additional sources of data that may hold valuable information to identify predictors or provide context to the insights derived from the research. Likely data sources, such as social media, web traffic, and internal data siloed within an organization’s business areas, are candidates for linkage. The increasing availability of machine learning and advanced analytics tools capable of uncovering new insights is driving the push to identify a storage solution that can bring all of this data together.
Recognizing the need to bring data together, which data access model should you pursue?
Data Warehouses rely on an organized, typically relational database architecture. Warehouses use a schema-on-write methodology in which data is cleaned and formatted for predefined uses and stored in a file structure ready to be queried by various business applications. Due to the work involved in analyzing data sources and understanding how they relate to various business processes, warehouses generally consist of operational and transactional data, leaving out non-traditional and unstructured data. This upfront organization enables fast queries by Business Intelligence applications and more traditional operational dashboards intended for business units to monitor and analyze data over time. Due to the heavy investment required for data organization, a challenge of data warehouses is that they can be slow to adapt as new data sources become available.
Since data can exist in a lake without a predetermined business purpose, data is less likely to be “ignored” and therefore, there is greater opportunity for new insights through data exploration, machine learning, and predictive analytics.
Data Lakes use a schema-on-read approach, meaning data is applied to a plan or schema as it is pulled out of a stored location, rather than as it goes in. Because there is no upfront requirement to analyze and pre-define the relationships, data lakes can include anything from traditional transactional and operational data, to research data, syndicated data, log files, social media, images, audio, video, and more. Any stream of data is accepted. Structure is only applied after a use for the data is identified.
Since data can exist in a lake without a predetermined business purpose, data is less likely to be “ignored” and therefore, there is greater opportunity for new insights through data exploration, machine learning, and predictive analytics. Data lakes, however, run the risk of becoming unmanageable data swamps if deliberate care is not taken when selecting the data to add. Additionally, while a full schema does not need to be defined, some partitioning and defining of metadata should be applied to each stream feeding into the lake to help facilitate future searches, automated processes, and analyses.
Since data lakes can complement your existing structure, you don’t need to scrap your existing structure to get started. While the best fit will vary by organization, it is comforting to know that you don’t have to choose between data warehouses and data lakes.
James Dixon, who is widely attributed with coining the term “data lake,” suggests that data warehouses and lakes can, and likely should, coexist. With the warehouse, end-users define the questions they want to ask of the data, including the attributes required to answer those questions. That data is then loaded into data marts for consumption by the various business applications. This method works fine until you have a new question to ask. According to Dixon, you can solve this problem by incorporating a data lake alongside a warehouse:
Organizations require integration from numerous data sources stitched together to obtain superior insights.
“You store all of the data in a Data Lake, populate data marts and your data warehouse to satisfy traditional needs, and enable ad-hoc query and reporting on the raw data in the Data Lake for new questions.”
Many organizations already have an existing data warehouse structure or intentions to move existing business unit data silos into a company-wide system with the organization and reporting speed capabilities of a warehouse. With the increasing availability of non-traditional data and a demand for leveraging this data to drive business decisions, organizations require integration from numerous data sources stitched together to obtain superior insights.
This is the perfect opportunity for an organization to begin building a data lake. Refine and improve the traditional data storage and reporting structures that you have and enhance them with new insights pulled out of a data lake. It’s OK, and in fact advisable, to start small. The point is to get started. Start bringing that data together and benefiting from the new insights that you will gain!
As Director of Data Solutions, Neil serves in a senior advisory role for the company’s preparation and processing of data for analysis, contributes to the planning and execution of projects and oversees much of the internal and external training for our market research data processing and tabulation software packages.
As always, you can follow Burke, Inc. on our LinkedIn, Twitter, Facebook and Instagram pages.
Sources: Feature image (©Phongphan Supphakank – stock.adobe.com)