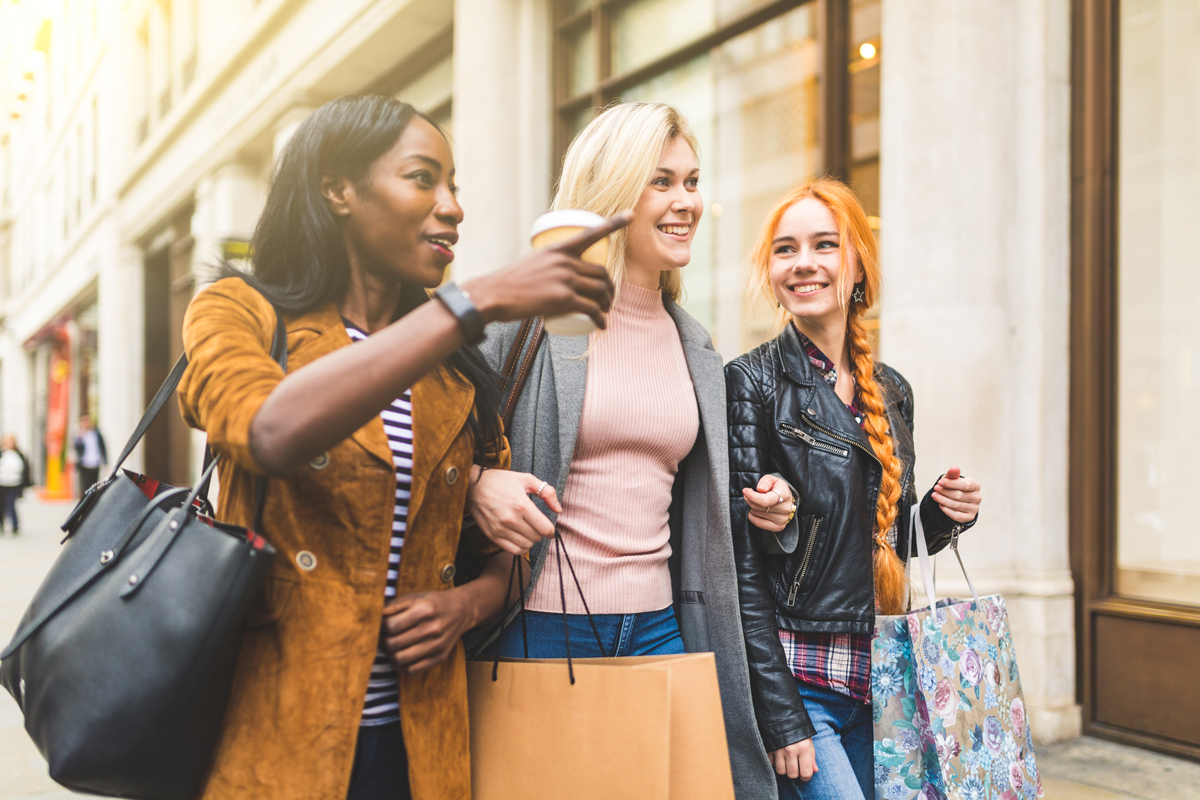
What are Conjoint Analysis and Discrete Choice Analysis?
Almost all human behaviors involve choice. And for any business that’s selling a product or service (which is most of them), understanding what drives a consumer’s choice to buy one thing vs. another is the essence of building a successful brand with relevant propositions. So, to help businesses understand consumer purchase decisions when faced with so many different options for products or services, market researchers often turn to Conjoint Analysis and Discrete Choice Analysis (C/DC) methodologies to uncover what’s really driving the choices consumers make.
The underlying assumption behind these trade-off analysis methods is that consumers make decisions based on what offers them the greatest utility. We can define “utility” as the overall relative importance of a potential choice (to a consumer) based on the unique combination of features/attributes that the choice offers. And since no two products or services are exactly alike in terms of their attribute values (e.g., brand, price, etc.), the total utility that a consumer derives from each product or service is also different. So, to calculate the utility of potential product/service choices, market researchers use a survey process that presents different combinations of features/attributes for respondents to choose between—this provides the basis for both Conjoint and Discrete Choice modeling.
Important Terminology
In Conjoint Analysis and Discrete Choice Analysis, each product or service has a “profile,” which consists of various “attributes.” Attributes are essentially like categories—color, size or brand, etc. Then within each attribute, you have “levels” that represent the different variables consumers might choose.
For example, automobile shoppers may be asked to evaluate what car they would choose based varying options of color, horsepower, storage/capacity levels, and brand options. In this example, product features like color would be known as “attributes,” while the different unique options within each attribute, such as blue or red or silver, are known as “levels.”
The list of attributes and levels reflects the business objectives and learning goals of the client; in other words, they want to understand how these specific things (i.e., attributes and levels) are impacting their consumers’ purchase behavior. The survey process enables consumers to evaluate these attributes by selecting the levels that appeal to them most. Collectively, the different attributes—and the levels within them—are known as the “choice set” of a conjoint analysis or discrete choice analysis.
Market researchers then use some pretty nifty choice modeling techniques and statistical tools to calculate the relative importance—or utility—of each unique level of each specific attribute being tested. Then, we can project the ideal product profile based on the levels with the highest-rated utility to consumers.
How can we use Conjoint and Discrete Choice analysis?
Conjoint and discrete choice analyses provide uniquely-detailed insights that can be used for the following strategic applications:
- Product Optimization and Innovation – Identifying the relative importance of key product attributes and levels that will have the biggest impact to increase consumers’ purchase likelihood.
- Market Simulation – Assessing market share based on more realistic estimates of preferences for various product configurations available to consumers.
- Segmentation – Determining different tranches of the marketplace by using respondent-level utilities to segment consumers based on their common product needs.
Conjoint analysis and discrete choice analysis can also be used dynamically—in conjunction with a web or Excel-based simulator that allows the user to test different market scenarios envisioned in the research objectives—in various tactical applications:
- Determining the value of specific attributes such as brand or price based on the relative importance of the attributes.
- Establishing the price for a product or service that optimizes purchase likelihood.
- Determining the impact of—and options for—responding to new competitive products or actions.
- Testing various scenarios and assessing how market share may change with the introduction of new and hypothetical product feature combinations.
A Closer Look at the Terms “Conjoint” and “Discrete Choice” Analysis
Traditional conjoint analysis works by allowing respondents to evaluate each product choice (i.e., attributes and levels) independently of the others to obtain the relative importance of each variable. The discrete choice model, on the other hand, presents several product profiles at once, with each profile being a unique combination of attribute levels. Respondents are then asked to choose between the different alternatives.
Because it presents choice as the outcome of interest within a competitive context, Discrete Choice exercises more closely resemble the real world where consumers often need to weigh more pros and cons in each scenario they’re considering. While there are cases where traditional Conjoint Analysis is suitable for a particular business objective—especially when competitive alternatives/substitutes do not exist—most situations call for Discrete Choice modeling. Indeed, nowadays, the terms Conjoint Analysis and Discrete Choice Analysis are often used interchangeably. But, in reality, the approach to research and analysis used is most typically Discrete Choice Analysis.
The Choice Set
A well-considered choice set is the foundation of all good discrete choice models. Because discrete choice models, in essence, simulate consumers’ decision-making processes among a set of options/alternatives in product features, presenting relevant, realistic choices is absolutely critical to the validity and reliability of the model.
In general, a good choice set of product features for conjoint analysis or discrete choice modeling must conform to a couple of underlying assumptions:
- The product features and levels contained in the choice set must be mutually exclusive from the survey respondents’ perspective. In other words, it must be clear to respondents (in a conjoint/discrete choice exercise) that by choosing one set of alternatives, s/he forgoes the possibility of the alternatives.
- Additionally, the choice set presented to the respondents should be considered exhaustive. That is to say, the options and alternatives that make up the choice set should contain all the relevant attributes and levels that make up the consumers’ universe of possibilities and determinants of choice.
In practice, the choice set in conjoint and discrete choice exercises are meticulously fine-tuned to fit the business and research objectives and conform to model assumptions. For example, one frequent adjustment to a discrete choice design entails forbidding certain combinations of attribute levels that are unrealistic or unavailable in the marketplace (say, a premium combination of features that carry a very low price). In addition, a key caution for any conjoint or discrete choice design is the accidental omission of a determinative attribute or level—in other words, one that has a major impact on respondent preference. Such omissions may become latent factors that adversely impact the accuracy of resulting model parameters and the actionability of the results.
Final Words on Research Process and Model Estimation
Once we have a well-designed conjoint or discrete choice model choice set (which is often accomplished via co-creation between clients who are intimately familiar with their products and researchers who are methodological experts), we have the basis of a good model. From there, research and analytic experts (such as Burke) will determine the exact manner in which the scenarios are presented to respondents (say, how many alternatives we show each respondent, whether a “none” option is included, etc.).
As for the specific model forms used in traditional conjoint analysis and discrete choice modeling, several common analytic techniques include: logit, nested logit, mixed logit, probit models (with corresponding methods of estimation using maximum likelihood), general method of moment, Bayesian, or simulation-based approaches. The underlying market dynamics and response pattern will dictate what analytic technique best fits the data, allowing analysts to derive realistic estimates for the underlying “random utility function” and market share estimation.
With the requisite level of forethought in terms of design and execution, Conjoint and Discrete Choice research serve as powerful tools for optimizing product and service offerings, especially when used with an Excel-based simulator. Whether realigning existing propositions as markets change or vetting/validating a range of new offerings, these versatile methodologies provide a vital resource for marketers seeking to improve their probability of success.
If you’re interested in learning more about conjoint analysis and discrete choice reach out to us at info@burke.com.
As always, you can follow Burke, Inc. on our LinkedIn, Twitter, Facebook and Instagram pages.
Source: Feature Image – ©_KUBE_- stock.adobe.com